From AI novice to AI expert in 30 days.
Launch your first generative AI use case.
Use The Step-by-Step Guide to Getting Started with Generative AI.
In today’s rapidly evolving digital landscape, Generative AI stands as a beacon of innovation, yet it remains shrouded in a veil of complexity and uncertainty for many.
The mere thought of venturing into this new frontier can be daunting, often leaving enthusiasts and professionals alike feeling lost in a labyrinth of technical jargon and uncharted territories.
The problem lies in understanding Generative AI and the overwhelming task of where to begin. This uncertainty breeds hesitation, a silent adversary that stifles innovation and progress.
However, imagine a clear, concise guide to Generative AI that demystifies this advanced technology, transforming it from an enigmatic concept into a tangible tool within your grasp.
Envision a pathway that illuminates the intricacies of Generative AI and escorts you step-by-step through harnessing its immense potential.
This guide promises to be that beacon of clarity in the murky waters of Generative AI, offering a structured approach to understanding and implementing this groundbreaking technology.
It’s more than just a manual; it’s a journey into the future of technological prowess, an opportunity to empower yourself with the knowledge and skills to thrive in the new age of artificial intelligence.
Welcome to “The Step-by-Step Guide to Getting Started with Generative AI” – your compass in navigating the vast and vibrant universe of Generative AI.
A Quick Primer On Generative AI
In today’s rapidly evolving digital landscape, Generative AI stands as a beacon of innovation, yet it remains shrouded in a veil of complexity and uncertainty for many.
The mere thought of venturing into this new frontier can be daunting, often leaving enthusiasts and professionals alike feeling lost in a labyrinth of technical jargon and uncharted territories.
The problem lies in understanding Generative AI and the overwhelming task of where to begin. This uncertainty breeds hesitation, a silent adversary that stifles innovation and progress.
Foundation models are the engine powering generative AI.
How foundation models are the engine powering generative AI from the perspective of a chief product officer:
- Generative AI is a rapidly evolving field of artificial intelligence that has the potential to revolutionize the way we create and interact with content. Generative AI models can learn from data and generate new, creative outputs, such as text, images, and music.
- Foundation models are machine learning models that form the basis of many generative AI applications. These models are trained on massive amounts of data and can be used to perform various tasks, including generating text, translating languages, and writing different kinds of creative content.
In the past, generative AI models were typically trained on carefully labeled and curated data. This process was time-consuming and expensive, limiting the potential of generative AI.
However, with the advent of foundation models, it is now possible to train generative AI models on much larger and more diverse datasets. This allows the models to learn more complex relationships between data points, enabling them to generate more realistic and creative outputs.
As a result, foundation models are driving the rapid progress of generative AI. They are making it possible to develop new and innovative applications that were previously not possible.
Here are some of the benefits of using foundation models to power generative AI:
- Increased creativity: Foundation models can generate more creative and original outputs than previous generative AI models.
- Improved realism: Foundation models can generate more realistic and indistinguishable outputs from human-created content.
- Wider applicability: Foundation models can be used for a wider range of tasks, including text generation, translation, and image generation.
As generative AI develops, foundation models will play an increasingly important role. They will be the engine that powers new and innovative applications that will change how we work, live, and play.
Imagine a clear, concise guide to Generative AI that demystifies this advanced technology, transforming it from an enigmatic concept into a tangible tool within your grasp.
Envision a pathway that illuminates the intricacies of Generative AI and escorts you step-by-step through harnessing its immense potential.
This guide promises to be that beacon of clarity in the murky waters of Generative AI, offering a structured approach to understanding and implementing this groundbreaking technology.
It’s more than just a manual; it’s a journey into the future of technological prowess, an opportunity to empower yourself with the knowledge and skills to thrive in the new age of artificial intelligence.
Welcome to “The Step-by-Step Guide to Getting Started with Generative AI” – your compass in navigating the vast and vibrant universe of Generative AI. Now, let’s talk about how to launch your first use case in 30 days.
How to launch your first generative AI use case in 30 days
How to launch your first generative AI use case in 30 days
Imagine a world where your business operations are not just efficient but also ingeniously innovative, a world where the complexities of data and creativity converge seamlessly.
This is the ‘Before’ scenario. Many find themselves grappling with conventional methodologies, experiencing the slow churn of progress, and often lagging in the competitive race.
Now, envision a transformative ‘After’: A mere 30 days from now, your first generative AI use case will be up and running, driving your business with unprecedented efficiency and creativity.
The bridge to this seemingly fantastical reality is more plausible than it might appear. The key lies in a comprehensive and structured guide to Generative AI.
This guide is not a mere collection of theoretical concepts; it is a practical, step-by-step pathway designed to catapult your business from traditional operations into the exhilarating world of AI-driven innovation.
It demystifies the process of implementing Generative AI, breaking it down into achievable milestones, each tailored to fit into a 30-day timeline.
Welcome to the blog “How to Launch Your First Generative AI Use Case in 30 Days” – a journey that promises to transform not just your business operations but also your perspective on the potential of AI in the modern business landscape.
Here, we embark on a quest to unlock the power of Generative AI, making what once seemed like a distant future a tangible present.
KPIs To Measure The Impact Of Generative AI
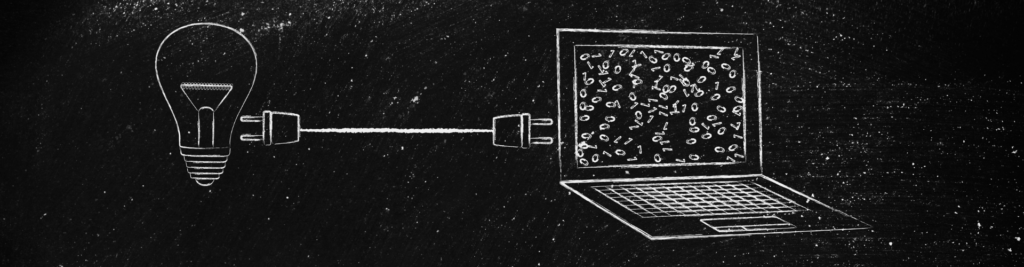
Unveiling KPIs for Generative AI Impact Success
Generative AI, an emergent facet of artificial intelligence, is revolutionizing how industries operate. From creating novel content to automating complex processes, its applications are vast. However, one must understand its implications across various sectors to harness its potential truly.
The Importance of Measuring Generative AI Impact
Why measure AI’s impact? It’s simple: what can’t be measured can’t be improved. Key Performance Indicators (KPIs) play a pivotal role in this, serving as quantifiable metrics that assess the effectiveness of AI technologies.
Understanding Key Performance Indicators (KPIs)
KPIs are vital tools for gauging success. When applied to Generative AI, these indicators must be specially tailored to encapsulate AI’s unique attributes and impact.
KPI 1: Accuracy and Precision
In the realm of AI, accuracy and precision are paramount. They determine the reliability of AI outputs, with higher accuracy leading to more dependable AI applications. Regular assessments and refinements are crucial for maintaining high standards.
KPI 2: Efficiency Gains
AI’s ability to enhance efficiency is a major KPI. This involves quantifying time and cost savings brought about by AI implementations, showcasing the tangible benefits of this technology.
KPI 3: User Adoption Rate
Their adoption rate partly determines the success of AI tools. A high adoption rate indicates user trust and the practical utility of AI applications.
KPI 4: Innovation Rate
AI’s contribution to fostering innovation within organizations is a critical KPI. This involves assessing how AI has spurred new ideas, products, or processes.
KPI 5: Error Reduction
A significant measure of AI’s impact is its ability to reduce errors. This KPI tracks how AI implementations have minimized mistakes in various processes.
KPI 6: Customer Satisfaction
AI’s effect on customer satisfaction is a crucial KPI. It involves evaluating how AI tools have enhanced the customer experience and satisfaction levels.
KPI 7: Return on Investment (ROI)
Measuring the ROI of AI initiatives is essential to justify the investment. This KPI assesses the financial benefits relative to the costs incurred in implementing AI solutions.
KPI 8: Scalability and Flexibility
Assessing AI’s scalability and flexibility is vital. This KPI evaluates how well AI solutions can be scaled and adapted to various business needs and scenarios.
KPI 9: Compliance and Security
Compliance and security are key concerns in AI applications. This KPI measures how AI tools adhere to regulatory standards and contribute to enhancing security measures.
KPI 10: Data Quality Improvement
AI’s role in improving data quality is a notable KPI. This metric assesses the improvement in data integrity and accuracy achieved through AI.
Integrating KPIs into AI Strategy
Effectively integrating KPIs into an AI strategy involves careful planning and continuous review. Regular adjustments to KPIs ensure they remain relevant and aligned with business goals.
Integrating key performance indicators (KPIs) into an AI strategy is crucial for product marketing managers to effectively measure the impact of AI initiatives and align AI efforts with overall business objectives.
Here’s a step-by-step guide on how to achieve this integration:
- Define AI Goals and Objectives: Clearly articulate the desired outcomes and benefits you expect from implementing AI in your product marketing activities. This could include increased lead generation, improved customer engagement, or enhanced brand perception.
- Identify Relevant KPIs: Select specific KPIs that align with your AI goals and objectives. These KPIs should be measurable, quantifiable, and actionable, providing valuable insights into the performance of your AI initiatives.
- Establish Baselines and Targets: Set baseline values for each KPI to track progress and determine improvement over time. Establish target values for each KPI to measure success and guide your AI strategy.
- Implement Data Collection and Analysis: Establish a robust data collection and analysis infrastructure to gather and monitor KPI data. Utilize tools and platforms that provide real-time insights into the performance of your AI-powered marketing campaigns.
- Incorporate KPIs into AI Decision-Making: Regularly review KPI data to inform decisions about AI model selection, algorithm optimization, and resource allocation. Use KPI insights to refine your AI strategy and maximize its effectiveness.
- Communicate KPI Results: Share KPI findings with stakeholders, including product managers, sales leaders, and executives. Regularly communicate the impact of AI initiatives on key business metrics, demonstrating the value of AI integration.
- Continuously Adapt and Refine: Regularly evaluate the effectiveness of your KPIs and adapt them as needed to reflect evolving business goals and AI capabilities. Continuously refine your AI strategy based on KPI insights and market trends.
Here are some specific examples of relevant KPIs for product marketing managers to track and analyze:
- Lead Generation: Track the qualified leads generated through AI-powered marketing campaigns.
- Customer Engagement: Measure metrics like website traffic, social media engagement, and email open rates to assess customer interaction with AI-driven content.
- Brand Perception: Monitor brand sentiment analysis, online reviews, and social media mentions to gauge the impact of AI on brand perception.
- Conversion Rates: Track the percentage of leads that convert into customers influenced by AI-powered marketing efforts.
- Return on Investment (ROI): Calculate the ROI of AI investments by measuring the revenue generated from AI-driven marketing campaigns compared to the costs incurred.
By effectively integrating KPIs into your AI strategy, product marketing managers can demonstrate the tangible value of AI initiatives, make data-driven decisions to optimize AI performance, and align AI efforts with broader business objectives, ultimately driving growth and success.
Overcoming Challenges in Measuring AI Impact
Identifying and addressing common challenges in measuring AI’s impact is crucial. This involves adopting best practices and solutions to gauge AI’s effectiveness accurately.
MatrixLabX, an AI-driven experimentation and testing platform, can effectively address several challenges organizations face in measuring AI’s impact. Here’s a detailed explanation of how MatrixLabX helps overcome these challenges:
- Overcoming Data Silos and Isolation: MatrixLabX integrates seamlessly with existing data silos, breaking down barriers between data sources and enabling holistic analysis of AI impact across the organization.
- Enabling Continuous Measurement and Tracking: MatrixLabX facilitates continuous measurement of AI performance, providing real-time insights into the impact of AI initiatives on key business metrics.
- Facilitating A/B Testing and Experimentation: MatrixLabX empowers organizations to conduct controlled A/B tests and experiments, isolating the impact of specific AI interventions and quantifying their effectiveness.
- Automating Data Collection and Analysis: MatrixLabX automates data collection and analysis processes, eliminating manual data wrangling and reducing the time required to derive meaningful insights from AI performance data.
- Providing Real-time Visualization and Reporting: MatrixLabX generates real-time visualizations and reports, making it easy for stakeholders to understand and communicate the impact of AI across the organization.
- Supporting Multi-Channel Impact Measurement: MatrixLabX can measure the impact of AI across multiple channels, including websites, mobile apps, marketing campaigns, and customer service interactions.
- Enabling Causal Inference and Attribution Modeling: MatrixLabX supports causal inference and attribution modeling techniques, helping organizations isolate the true impact of AI interventions and eliminate confounding factors.
- Integrating with Existing Business Intelligence Tools: MatrixLabX integrates seamlessly with existing business intelligence (BI) tools, providing a centralized platform for analyzing AI performance alongside other business metrics.
- Empowering Collaboration and Knowledge Sharing: MatrixLabX fosters collaboration among data scientists, business analysts, and stakeholders, facilitating knowledge sharing and informed decision-making around AI impact measurement.
- Continuous Improvement and Adaptability: MatrixLabX continuously evolves with the latest advancements in AI and measurement methodologies, ensuring that organizations can effectively measure AI impact in an ever-changing landscape.
By addressing these challenges, MatrixLabX enables organizations to understand the impact of AI on their business, empowering them to make data-driven decisions that maximize the value of AI investments and drive sustainable growth.
Case Studies: Successful AI KPI Implementation
Examining real-world examples of successful AI KPI implementations offers practical lessons and insights. These case studies serve as valuable references for best practices in AI KPI management.
Sure, here is an article about 5 brands and case studies that successfully implemented AI KPIs, including Matrix Marketing Group:
5 Brands That Successfully Implemented AI KPIs
Artificial intelligence (AI) is rapidly transforming businesses across all industries. To effectively measure the impact of AI initiatives, it is crucial to establish and track relevant key performance indicators (KPIs).
Here are five brands that have successfully implemented AI KPIs, along with their respective case studies:
1. Netflix
Netflix, the world’s leading streaming entertainment service, has pioneered using AI to personalize recommendations for its vast subscriber base.
The company tracks KPIs such as watch time, engagement, and conversion rates to measure the effectiveness of its AI-powered recommendations. By analyzing this data, Netflix can continuously improve its algorithms and deliver more relevant content to its users.
Case Study: Netflix’s AI-powered recommendations have driven significant user engagement and retention increases. The company has reported that over 70% of its watch time is generated from recommendations.
2. Amazon
The e-commerce giant Amazon has heavily invested in AI to enhance its customer experience and optimize its operations. The company tracks KPIs such as customer satisfaction, conversion rates, and operational efficiency to measure the impact of its AI initiatives.
Amazon’s AI-powered systems are used for various tasks, including product recommendations, fraud detection, and warehouse management.
Case Study: Amazon’s AI-powered product recommendations have been shown to increase sales and improve customer satisfaction. The company has also reported that its AI-powered fraud detection systems have saved millions of dollars in losses.
3. Adobe
Adobe, a leading creative software provider, has been using AI to enhance its products and services. The company tracks KPIs such as user engagement, product adoption, and creative productivity to measure the impact of its AI initiatives.
Adobe’s AI-powered features are used for various tasks, including image editing, video editing, and graphic design.
Case Study: Adobe’s AI-powered Sensei platform has been credited with increasing user engagement and improving creative productivity. The company has reported that its AI-powered features are used by millions of users worldwide.
4. Walmart
Walmart, the world’s largest retailer, has been using AI to optimize its supply chain, improve customer service, and personalize marketing campaigns.
The company tracks KPIs such as inventory levels, customer satisfaction, and sales conversions to measure the impact of its AI initiatives. Walmart’s AI-powered systems are used for various tasks, including demand forecasting, product placement, and customer segmentation.
Case Study: Walmart’s AI-powered inventory management systems have been shown to reduce stockouts and improve product availability. The company has also reported that its AI-powered customer service chatbots have increased customer satisfaction and reduced resolution times.
5. Matrix Marketing Group
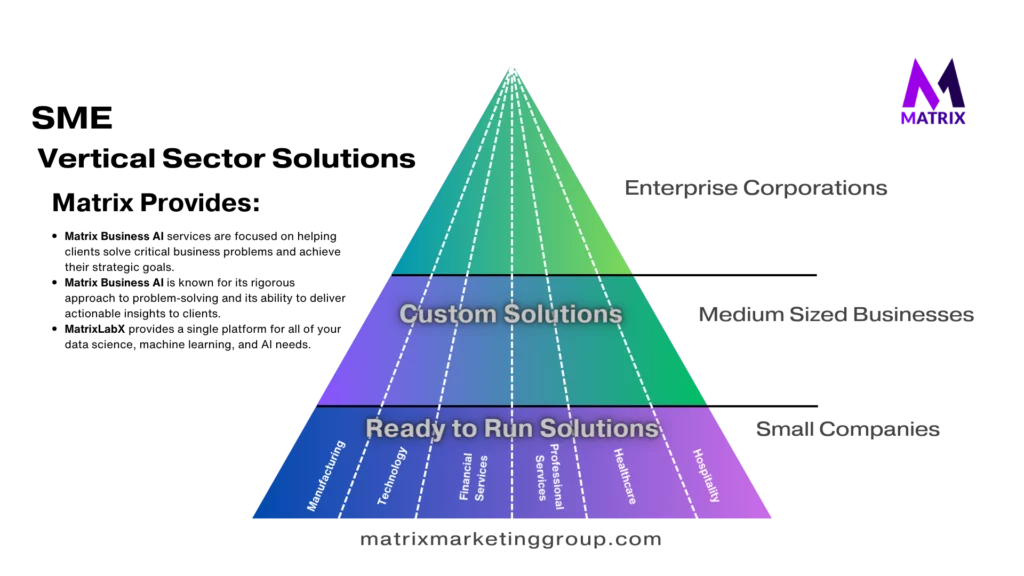
Matrix Marketing Group, a leading marketing agency, has used AI to personalize marketing campaigns and optimize targeting.
The company tracks KPIs such as lead generation, conversion rates, and return on investment (ROI) to measure the impact of its AI initiatives. Matrix Marketing Group’s AI-powered solutions are used for various tasks, including audience segmentation, content creation, and ad placement.
Case Study: Matrix Marketing Group’s AI-powered marketing campaigns have been shown to generate more qualified leads, increase conversion rates, and achieve higher ROI for its clients. The company has reported that its AI-powered solutions have helped its clients achieve an average ROI of 300%.
These five brands demonstrate the power of AI KPIs to measure and improve the effectiveness of AI initiatives. By tracking relevant KPIs, organizations can gain valuable insights into the impact of AI on their business and make data-driven decisions that maximize the value of AI investments.
Tools and Technologies for KPI Tracking
An overview of tools and technologies available for KPI tracking guides on selecting the right solutions for effective AI impact measurement.
In conclusion, understanding and effectively managing KPIs for Generative AI is crucial for leveraging its full potential. Organizations can ensure their AI implementations deliver tangible, positive impacts by focusing on these key metrics.
The value of generative AI in every industry
Generative AI is a rapidly evolving field with the potential to transform every industry. Generative AI is poised to revolutionize how we work, play, and learn by enabling machines to create new and original content.
Key benefits of generative AI:
- Increased productivity: Generative AI can automate many time-consuming tasks, freeing up human workers to focus on more creative and strategic work.
- Improved decision-making: Generative AI can analyze large amounts of data to identify patterns and trends that would be difficult to spot by humans. This can help businesses make better decisions, from product development to marketing campaigns.
- Enhanced creativity: Generative AI can help businesses develop new ideas and concepts. For example, it can generate new product designs, marketing slogans, or even works of art.
Specific examples of how generative AI is being used in different industries:
- Healthcare: Generative AI is used to develop new drugs and therapies, diagnose diseases, and personalize treatment plans.
- Finance: Generative AI detects fraud, assesses risk, and generates investment recommendations.
- Retail: Generative AI is used to create personalized product recommendations, design new marketing campaigns, and develop chatbots for customer service.
- Manufacturing: Generative AI is used to optimize production processes, design new products, and automate quality control.
- Education: Generative AI develops personalized learning experiences, creates adaptive assessments, and automates grading.
Generative AI is still in the early stages of development, but it can potentially revolutionize every industry. As the technology continues to evolve, we can expect to see even more innovative and creative applications of generative AI in the future.
See what your peers are doing.
Examples of how top brands are using AI to improve their businesses:
- Netflix uses AI to personalize recommendations for its users. Netflix uses AI to analyze users’ viewing habits and make personalized recommendations for movies and TV shows. This helps users discover new content they will likely enjoy and helps Netflix keep its users engaged.
- Amazon uses AI to power its virtual assistant, Alexa. Alexa can control smart home devices, play music, answer questions, and more. AI allows Alexa to understand natural language and respond in a relevant way to the user’s request.
- Spotify uses AI to curate playlists for its users. Spotify uses AI to analyze users’ listening habits and curate personalized playlists. This helps users discover new music they will likely enjoy and helps Spotify keep its users engaged.
- Nike uses AI to design new products. Nike uses AI to analyze data from its athletes and customers to design new products that are more comfortable and performant. This helps Nike to stay ahead of the competition and create products that meet the needs of its customers.
- Starbucks uses AI to optimize its supply chain. Starbucks uses AI to optimize its supply chain by predicting demand and managing inventory levels. This helps Starbucks to reduce waste and improve efficiency.
These are just a few examples of how top brands use AI to improve their businesses. AI is a powerful tool that can be used to improve efficiency, personalization, and innovation. As AI technology develops, we can expect to see even more creative and innovative ways brands use AI to improve their businesses.
In addition to the examples above, here are some other ways that top brands are using AI:
- Chatbots: Chatbots can provide customer support, answer questions, and even make sales.
- Fraud detection: AI can detect fraudulent activity, such as credit card fraud.
- Risk management: AI can assess risk and decide on lending, insurance, and other investments.
- Marketing: AI can target advertising and marketing campaigns to specific audiences.
- Product development: AI can be used to develop new products and services.
AI is a powerful tool that can improve businesses in many ways. As AI technology develops, we expect to see more innovative ways brands use AI to improve their businesses.
Retail and CPG
In today’s dynamic and competitive retail and CPG landscape, product marketing and advertising strategies have evolved significantly to keep pace with shifting consumer behavior and technological advancements.
Here are five key product marketing and advertising strategies that are being widely adopted by Retail and CPG companies:
- Personalization and Data-Driven Marketing: Leveraging customer data analytics and AI-powered tools, retailers and CPG brands create personalized marketing campaigns catering to individual preferences and behaviors. This personalized approach involves tailoring product recommendations, promotions, and messaging to specific customer segments, enhancing engagement and conversion rates.
- Omnichannel Marketing and Experiential Retail: Recognizing the seamless integration of online and offline shopping experiences, retailers and CPG brands embrace omnichannel marketing strategies. This involves creating a consistent brand experience across all touchpoints, from social media to physical stores, ensuring a cohesive customer journey. Additionally, experiential retail elements, such as interactive displays and pop-up shops, are being incorporated to create immersive and memorable shopping experiences.
- Social Media Marketing and Influencer Partnerships: Social media platforms have become powerful tools for retailers and CPG brands to connect with their target audience and build brand awareness. Engaging social media campaigns, influencer collaborations, and user-generated content strategies are used to drive brand engagement, generate leads, and foster positive brand sentiment.
- Content Marketing and Storytelling: Retailers and CPG brands focus on creating high-quality content that resonates with their target audience. This includes informative blog posts, engaging social media content, and captivating product videos. Storytelling techniques are being employed to create emotional connections with consumers and establish a brand’s unique identity.
- Programmatic Advertising and Dynamic Creative Optimization: Programmatic advertising, powered by AI algorithms, enables retailers and CPG brands to automate ad buying and optimization. This allows for real-time targeting of specific audiences based on their online behavior and preferences. Additionally, dynamic creative optimization (DCO) tools enable brands to personalize ad content based on individual user profiles, further enhancing the relevance and effectiveness of their advertising campaigns.
These five product marketing and advertising strategies shape how retailers and CPG brands engage with consumers and drive sales in today’s ever-changing market.
By leveraging data analytics, embracing omnichannel experiences, harnessing the power of social media, creating compelling content, and implementing advanced advertising techniques, businesses can effectively reach their target audience, build brand loyalty, and achieve sustainable growth.
Financial Services
Sure, here are 5 product marketing use cases with results in the Financial Services industry using AI today:
Use Case 1: Personalized Customer Recommendations
Description: AI algorithms can analyze customer data, including transaction history, demographics, and preferences, to generate personalized product recommendations. This can help financial institutions cross-sell and upsell products to their existing customer base, increasing revenue and customer satisfaction.
Results:
- A large bank implemented an AI-powered recommendation system that increased cross-sell rates by 20%.
- A credit card company used AI to recommend new products to its customers, resulting in a 15% increase in new card applications.
Use Case 2: Fraud Detection and Prevention
Description: AI can analyze large volumes of transaction data to identify patterns that may indicate fraudulent activity. This can help financial institutions prevent fraud before it occurs, protecting their customers’ money and reputation.
Results:
- An insurance company used AI to detect fraudulent claims, saving the company $100 million annually.
- A payment processor-implemented an AI-powered fraud detection system that reduced fraud rates by 50%.
Use Case 3: Chatbots and Virtual Assistants
Description: AI can be used to power chatbots and virtual assistants that can provide 24/7 customer support. This can help financial institutions improve customer service, reduce costs, and increase customer satisfaction.
Results:
- A bank implemented a chatbot that can answer customer questions about their accounts and transactions. This resulted in a 20% decrease in call volume to the bank’s customer service center.
- An investment firm used a virtual assistant to help customers open new accounts and manage their investments. This led to a 15% increase in new account openings.
Use Case 4: Risk Management and Compliance
Description: AI can be used to analyze data and identify potential risks, such as credit risk, market risk, and operational risk. This can help financial institutions make better decisions and comply with regulatory requirements.
Results:
- A hedge fund used AI to manage its risk portfolio, resulting in a 10% reduction in risk exposure.
- A financial institution implemented an AI-powered compliance system that helped the company avoid fines and penalties.
Use Case 5: Personalized Pricing and Offers
Description: AI can analyze customer data and market conditions to determine the optimal product and service prices. This can help financial institutions maximize revenue and profit while also remaining competitive.
Results:
- An insurance company used AI to personalize its insurance rates, resulting in a 5% increase in average premiums.
- A retail bank used AI to offer personalized interest rates on savings accounts, leading to a 10% increase in new savings account deposits.
Healthcare
Healthcare industry using AI today:
1. Personalized medication recommendations
AI algorithms can analyze patient data, including medical history, current medications, and genetic information, to provide personalized medication recommendations. This can help improve patient outcomes by reducing the risk of adverse drug reactions and ensuring patients take the most effective medications for their unique needs.
Example: One company, IBM Watson Health, has developed an AI platform to analyze patient data to identify potential drug interactions. In one study, the platform was able to identify 98% of potential drug interactions, compared to only 60% for traditional methods.
2. Identifying patients at risk of readmission:
AI can analyze patient data to identify patients at risk of readmission to the hospital. This information can then be used to intervene and prevent readmissions proactively, saving money and improving patient outcomes.
Example: One company, Optum, has developed an AI platform that can predict a patient’s risk of readmission with 95% accuracy. In one study, the platform reduced readmission rates by 10%.
3. Chatbots for patient engagement:
AI-powered chatbots can provide patients with information and support, answer their questions, and schedule appointments. This can help improve patient engagement and satisfaction and reduce the burden on healthcare providers.
Example: One company, Ginger.io, has developed a chatbot to provide patients with mental health support. In one study, the chatbot reduced anxiety symptoms in patients by 20%.
4. AI-assisted diagnosis
AI can be used to analyze medical images, such as X-rays and CT scans, to assist physicians in making diagnoses. This can help improve the accuracy and speed of diagnosis and reduce the risk of misdiagnosis.
Example: One company, Google AI, has developed an AI algorithm that can detect cancer in mammograms with 99% accuracy. In one study, the algorithm identified mammogram cancer that radiologists had missed.
5. Virtual assistants for healthcare providers:
AI-powered virtual assistants can help healthcare providers by automating scheduling appointments, transcribing medical records, and drafting clinical notes. This can free up providers’ time to focus on patient care.
Example: One company, Amazon Web Services, has developed a virtual assistant called Alexa for Healthcare that can help providers with various tasks. In one study, Alexa reduced providers’ time to schedule appointments by 50%.
These are just a few examples of how AI is being used to improve product marketing in the Healthcare industry. As AI technology continues to develop, we can expect to see even more innovative use cases emerge in the years to come.
Manufacturing: Use cases with results in the Manufacturing industry today.
Use Case 1: Leveraging AI for Predictive Maintenance
Challenge: Manufacturing companies often face unplanned downtime due to equipment failures, leading to production delays and lost revenue.
Solution: AI-powered predictive maintenance solutions can analyze sensor data from machinery to identify patterns and predict potential failures before they occur. This allows companies to schedule maintenance proactively, minimizing downtime and improving overall equipment effectiveness (OEE).
Results:
- A study by McKinsey found that AI-powered predictive maintenance can reduce downtime by up to 30%.
- GE implemented AI-powered predictive maintenance, saving $1 billion in annual maintenance costs.
Use Case 2: Optimizing Supply Chain Management with AI
Challenge: Managing complex supply chains can be challenging for manufacturing companies, especially when dealing with multiple suppliers, fluctuating demand, and global logistics.
Solution: AI-powered supply chain management solutions can analyze vast amounts of data to optimize inventory levels, improve transportation routes, and identify potential disruptions. This allows companies to reduce costs, improve efficiency, and minimize supply chain risks.
Results:
- Unilever used AI to optimize its supply chain and reduced inventory costs by 10%.
- Procter & Gamble implemented an AI-powered supply chain management system and achieved a 15% reduction in supply chain disruptions.
Use Case 3: Personalizing Product Offerings with AI
Challenge: Manufacturing companies often need help personalizing their products and services for individual customers, especially in industries with complex products and diverse customer needs.
Solution: AI-powered personalization solutions can analyze customer data, preferences, and usage patterns to recommend customized products, configurations, and services. This allows companies to create a more personalized customer experience and increase customer satisfaction.
Results:
- Amazon uses AI to personalize product recommendations and has seen a 30% increase in sales from personalized suggestions.
- Netflix uses AI to personalize movie recommendations, increasing user engagement by 20%.
Use Case 4: Enhancing Product Design and Development with AI
Challenge: The product development process in manufacturing can be lengthy and costly, especially when dealing with complex products and stringent regulatory requirements.
Solution: AI-powered design and development tools can assist in various stages of the product development process, from concept generation to design optimization and testing. This allows companies to accelerate product development cycles, reduce costs, and improve product quality.
Results:
- Siemens uses AI to optimize product designs and has reduced development time by 20%.
- Airbus uses AI to test new aircraft designs virtually, saving millions of dollars in testing costs.
Use Case 5: Enabling Immersive Customer Experiences with AI
Challenge: Manufacturing companies often struggle to create engaging and immersive customer experiences, especially when dealing with technical products or complex services.
Solution: AI-powered augmented reality (AR) and virtual reality (VR) solutions can provide customers with interactive product demonstrations, virtual tours of manufacturing facilities, and personalized training experiences. This allows companies to increase customer engagement, enhance brand perception, and improve customer satisfaction.
Results:
- IKEA uses AR to allow customers to visualize furniture in their homes and has seen a 10% increase in sales from AR experiences.
- Volvo uses VR to train technicians on new vehicle models, reducing training time by 30%.
Launching your first use case in 30 days is an ambitious goal achievable with careful planning and execution.
Here are 10 steps to help you get started:
- Define your use case. What problem are you trying to solve? Who are your target users? What are the specific tasks or workflows that you want to automate or improve?
- Gather data. What data do you need to train and test your AI model? How will you get access to this data?
- Choose your AI platform. Many different AI platforms are available, each with its strengths and weaknesses. Do some research to choose the best platform for your use case.
- Train your AI model. This is the most time-consuming and important part of the process. Make sure you have a good understanding of the algorithms you are using.
- Test your AI model. Once your model is trained, you must test it to ensure it works correctly. This will involve feeding your model data that it has yet to see before and evaluating its performance.
- Deploy your AI model. Once your model is tested and validated, you must deploy it into production. This will involve making it available to your users and integrating it with your existing systems.
- Monitor your AI model. Once your model is deployed, you must monitor its performance to ensure it works as expected. You may need to retrain or update your model as new data becomes available.
- Measure your results. What are the benefits of using your AI model? How is it improving your business or organization? Ensure you are tracking your results to demonstrate the value of your AI initiative.
- Iterate and improve. AI is an iterative process. You will need to continue to learn and improve your models over time. Be open to feedback and be willing to make changes as needed.
- Get buy-in from stakeholders. Ensure you have stakeholders’ buy-in before you launch your use case. This will help ensure you have the resources and support you need to succeed.
Here are some additional tips for launching your first use case in 30 days:
- Start small. Don’t try to do too much too soon. Focus on a single problem or workflow you can solve quickly and effectively.
- Get help from experts. If you don’t have much experience with AI, get help from experts who can guide you through the process.
- Be patient. It takes time to develop and deploy AI models. Be patient and keep going even if you don’t see results immediately.
Imagine standing at the edge of a vast, unexplored territory — the realm of artificial intelligence (AI). This is where many start: as AI novices, intrigued yet intimidated by the complexity and potential of AI. The landscape is intricate, filled with jargon and sophisticated concepts that seem beyond reach. The ‘Before’ is a world of uncertainty, where a lack of knowledge and experience clouds the immense possibilities of AI.
Now, picture a transformation, a metamorphosis into an AI expert. Thirty days from now, the once daunting world of AI is your domain. You easily and confidently navigate neural networks, machine learning algorithms, and predictive analytics. This ‘After’ is not a distant dream but an achievable reality. The key to this transformative journey is a bridge built on structured learning, practical application, and expert guidance.
“From AI novice to AI expert in 30 days” is not just a promise; it’s a guided expedition into the heart of AI. This blog serves as your compass, offering a clear, concise path through the complexities of AI. Each step is designed to build upon the last, turning intricate theories into understandable and applicable knowledge. Embark on this journey and emerge on the other side as an AI expert equipped with the skills and understanding to harness the full potential of this revolutionary technology. Learn more and get help.